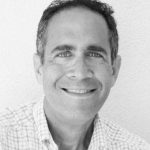
By DAVID SHAYWITZ, MD, PhD
As the nation wrestles with how best to return to normalcy, there’s a tension, largely but not entirely contrived, emerging between health experts—who are generally focused on maintaining social distancing and avoiding “preventable deaths”—and some economists, who point to the deep structural harm being caused by these policies.
Some, including many on the Trumpist-right, are consumed by the impact of the economic pain, and tend to cast themselves as sensible pragmatists trying to recapture the country from catastrophizing, pointy-headed academic scientists who never much liked the president anyway.
This concern isn’t intrinsically unreasonable. Most academics neither like nor trust the president. There is also a natural tendency for physicians to prioritize conditions they encounter frequently—or which hold particular saliency because of their devastating impact—and pay less attention to conditions or recommendations that may be more relevant to a population as a whole.
Even so, there are very, very few people on what we will call, for lack of a better term, “Team Health,” who do not appreciate, at least at some level, the ongoing economic devastation. There may be literally no one—I have yet to see or hear anyone who does not have a deep appreciation for how serious our economic problems are, and I know of a number of previously-successful medical practices which are suddenly struggling to stay afloat amidst this epidemic.
Continue reading…