By LUKE OAKDEN-RAYNER
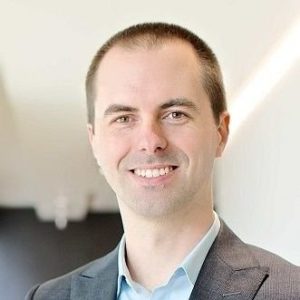
In the last post I wrote about the recent decision by CMS to reimburse a Viz.AI stroke detection model through Medicare/Medicaid. I briefly explained how this funding model will work, but it is so darn complicated that it deserves a much deeper look.
To get more info, I went to the primary source. Dr Chris Mansi, the co-founder and CEO of Viz.ai, was kind enough to talk to me about the CMS decision. He was also remarkably open and transparent about the process and the implications as they see them, which has helped me clear up a whole bunch of stuff in my mind. High fives all around!
So let’s dig in. This decision might form the basis of AI reimbursement in the future. It is a huge deal, and there are implications.
Uncharted territory
The first thing to understand is that Viz.ai charges a subscription to use their model. The cost is not what was included as “an example” in the CMS documents (25k/yr per hospital), and I have seen some discussion on Twitter that it is more than this per annum, but the actual cost is pretty irrelevant to this discussion.
For the purpose of this piece, I’ll pretend that the cost is the 25k/yr in the CMS document, just for simplicity. It is order-of-magnitude right, and that is what matters.
A subscription is not the only way that AI can be sold (I have seen other companies who charge per use as well) but it is a fairly common approach. Importantly though, it is unusual for a medical technology. Here is what CMS had to say:
Continue reading…