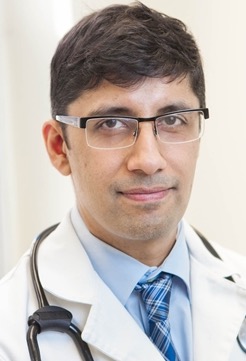
By ANISH KOKA, MD
The great pandemic is wreaking havoc, we are told, because the nation is not testing enough. The consensus from a diverse group that includes public health experts, economists, and silicon valley investors is that more testing will allow the country to restart the economy and do it safely.
The White House has been a mini laboratory for this testing strategy. Everyone who comes into contact with the President and Vice President is tested daily. This is supposedly what allows everyone to sit in meetings together and generally carry out the essential business of the country. But over this Mother’s Day weekend members of the White House spent their time scrambling to track down contacts of Katie Miller, the press secretary of the Vice president who tested positive. And contacts were left unclear about what exactly to do. One official started self-quarantining, while another did not.
If the White House has trouble with a mass testing, and contact tracing strategy, one wonders how this may work nationwide with thousands of new cases per day. While it would be tempting to blame administrative incompetence for the difficulties in the most important household in the land, the real difficulties lies with inherent limitations to tests that need to be understood before getting on the testing bandwagon.
RT-PCR
The type of test the world has been doing most often is based on knowing the genetic sequence of the RNA virus using a PCR test. PCR stands for Polymerase Chain Reaction, and it is used to directly detect the presence of the virus itself. The other type of test is an antibody test, used to detect the presence of disease fighting antibody proteins the body makes in response to attack from the virus and that persist for weeks, months or even years after the infection is over.
Standard PCR only works on DNA – the molecule that is the principal carrier of the genetic blueprint for humans. The COVID-19 virus uses RNA for its genome, which is similar but slightly different in structure from DNA. Luckily, viral enzymes to convert RNA into DNA have been discovered, so the PCR can be adapted to work on RNA in a process called reverse transcription PCR, or RT-PCR. Swabs are collected from parts of the body the virus is found and placed into a medium. In a lab, the RNA is extracted from the sample and then mixed with a cocktail that includes enzymes (DNA polymerase and reverse transcriptase), DNA building blocks, probes and short sequences of DNA called primers that have been specially designed to bind to this specific coronavirus.
The mixture is placed into a RT-PCR machine which cycles temperatures that trigger specific chemical reactions that create new, identical copies of the target section of the viral DNA. A typical RT-PCR machine goes through 35 cycles which translates to 34 billion new DNA copies for each RNA molecule. As new copies of the viral DNA sequence are being made, probes attached to specific target sequences are degraded by another enzyme which generates a fluorescent signal. A computer tracks the amount of fluorescence emitted, and when it reaches a sufficient level the virus is confirmed as present.
Serologic testing
Serologic testing identifies antibodies the body makes in response to the virus. Antibody identification involves taking a sample of usually blood and testing for the presence or absence of antibodies or measuring the amount of antibody present. Antibodies are proteins designed by the body to battle infections. Antibodies are unique, and selected to bind a specific part of the infectious pathogen which is called an antigen. Binding of antibodies to antigen results in a complex that attracts other cells in the body to kill the pathogen. Antibodies belong to a class of proteins known as Immunoglubulins. There are 5 different classes of immunoglobulins (IgM, IgG, IgE, IgA, IgD). IgM antibodies are produced in the initial response of the body to a virus or bacteria, while IgG antibodies are made later and represent a longer term response to guard against the return of the pathogen.
Detection of IgM antibodies represents an acute or recent infection, while detection of IgG antibodies suggests a past infection. Results can be reported as detected or not in a qualitative fashion (i.e., present or absent), or can be quantified in a measurement called antibody titers. Traditionally, antibody titers were determined using serial dilutions. The highest dilution that is still positive is reported, so a 1:320 dilution suggests a lot more antibody present than a 1:4 dilution.
One common method of testing for antibodies is the ELISA test (Enzyme Linked Immunosorbent Assay). In an ELISA, an antigen is immobilized on a solid surface. Fluid from blood is then added and antibodies against the antigen will then adhere to that antigen. A special antibody, usually from an animal source, that is linked to an enzyme that makes a signal such as a color change is used to confirm that antibodies against the antigen are present..
Lateral Flow Assays
Lateral flow assays (LFA) are low cost paper based platforms that offer simple, rapid and portable point of care detection devices. LFAs are tests that are widely used in hospitals and physician’s offices to allow detection of specific antigens, antibodies, and products of gene amplification. LFAs are cheap to produce, easy to use, and can be used with a variety of biological samples. For COVID, the specific type of test being discussed to detect antibodies is the Lateral flow Immunoassay (LFIA).
In this type of test, a liquid sample containing the material interest moves via capillary action through various zones on which molecules that can interact with the analyte are attached. The molecules attached to the paper strip are conjugated to coloured or fluorescent particles. If the sample has the analyte of interest, a response occurs on the test line. A response on the control line indicates adequate flow through the strip. LFIA is probably the most well known of all the tests because of its wide use in at home urine pregnancy tests.
Test considerations
A basic understanding of the accuracy of tests requires an understanding of four terms: Sensitivity, Specificity, Positive Predictive Value & Negative Predictive Value.
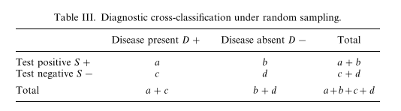
If one assumes the world can be divided into patients with disease and without disease using some gold standard test things become fairly easy. Validation of a test requires running on a test on a population with and without disease. For the purposes of this simple example, 100 patients known to have disease are tested with the new diagnostic test. If 90 of the 100 people with known disease test positive, this means 90 are true positives, and 10 are false negatives. Consequently, the test is said to be 90% sensitive. If the test is run on 100 people without disease, and 5 test positive, this means 95 are true negatives and 5 are false positives. This test is 95% specific. Sensitivity can be thought of as the true positive rate, and Specificity can be thought of as the true negative rate. Both are important for a test to be accurate.
What patients want to know, however, is what the chance of having a disease is depending on a test result. The probability of having the disease if testing positive is the positive predictive value. The probability of not having the disease if testing negative is referred to as the negative predictive value. The key point with predictive values is that they can’t be calculated without knowing the underlying prevalence of the disease.
As an example, let’s imagine an island of 1000 individuals with a prevalence of colon cancer of 1%. This means 10 patients have colon cancer on the island (0.01 x 1000). If we use the sensitivity and specificity from above (90% sensitive, 95% specific), 9 of the 10 patients are true positives, and 940 (0.95 x 990) patients are true negatives. This leaves 1 false negative, and 50 false positives.
Some simple math shows that a positive test in this case imparts a 15% probability of having the disease, while a negative test suggests a 99.8% probability of not having the disease.
Change the prevalence to 10% and the positive predictive value changes to 66%.
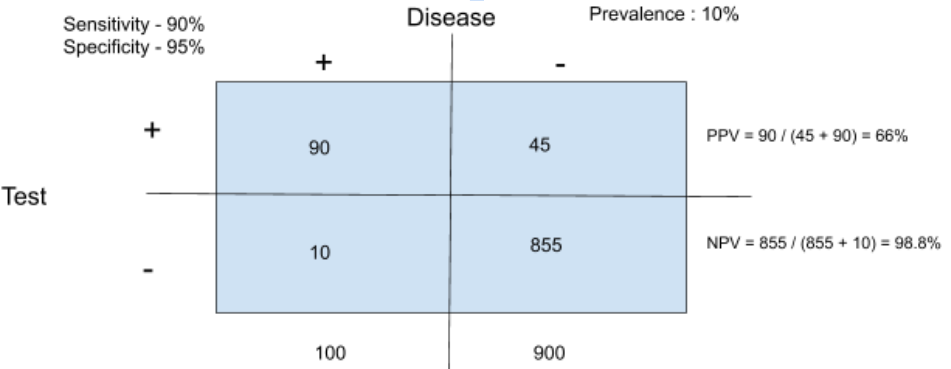
This still means that a test with 90% sensitivity and 95% specificity is poorly able to predict whether you have a given disease or not. Basically, if testing is taking place in a low prevalence population the test specificity has to be very high to be useful.
Graphed below is predictive values graphed over a range of prevalences (interactive shiny app here) for a test with 99% specificity. As is shown, even in a scenario with a much more specific test, the prevalence of disease in the population tested needs to be over 10% for the test to be useful to doctors and patients.
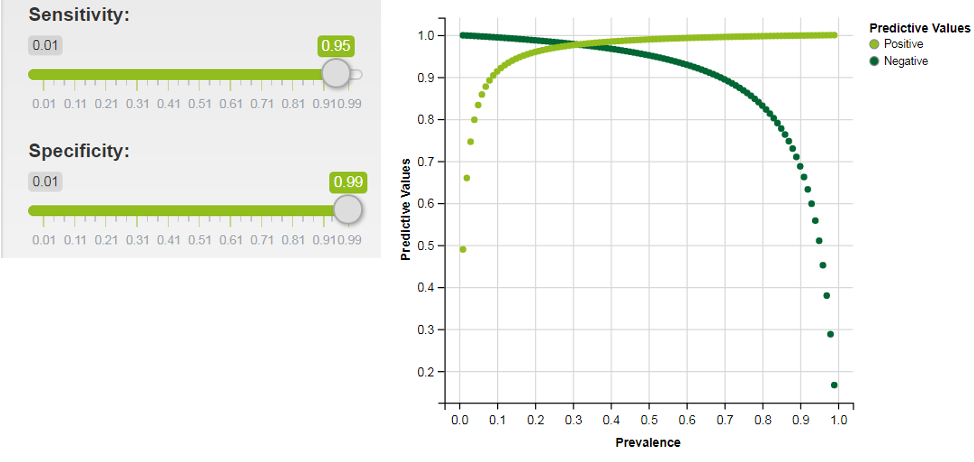
Specificity
Luckily RT-PCR is an incredibly specific test because the primers used are highly specific for the genetic information of interest. Unless the sample gets contaminated with genetic material from another COVID positive sample the test is essentially 100% specific. With that level of specificity, the positive predictive value is basically a 100% for a positive test. Unfortunately, serologic testing and lateral flow immunoassays are not as specific as PCR. SARS-COV2 belongs to the coronavirus family which has a number of related viruses that cause important diseases in humans. It is possible that antibody tests may cross-react to other non SARS-COV2 coronaviruses. Finding out the specificity of a test requires validation against samples that one “knows” to be negative. It may be impossible to be 100% certain about anything (more on that later) but in the case of SARS-COV2, there are a few approaches that may be taken. We can try to rely on judgement to decide who is negative by taking patients who have no symptoms, and have no recent known contact with the virus. Since it is believed SARS-COV2 may have relatively mild or no symptoms this risks the negative validation population being contaminated with patients that are actually positive. Another approach would be to take people with a recent confirmed infection with another coronavirus. This would be attractive because it would directly examine the tests ability to cross react with other coronaviruses, but it would also run the risk of including patients that may have had SARS-COV2 co-infections. The best approach is probably to use samples stored from a time prior to the onset of the current outbreak around the time of the year other coronaviruses are circulating, though even this is imperfect. We don’t know exactly when other coronaviruses circulate, and the antibody response to a circulating virus may wane over different periods of time. Clearly, this is very hard, and no test is going to be perfect.
Sensitivity
RT-PCR is unfortunately poorly sensitive. This has much to do with sample acquisition. The attempt to retrieve sample from the nasopharynx may not be successful because of inappropriate technique, patient discomfort, or low amounts of viral shedding at the time of testing. Reports of large numbers of false negatives suggest sensitivity ranges from 40-70% (1,2,3). Establishing sensitivity also has the same problems Specificity has: a gold standard. Known positive patients are the validation cohort. But barring another accepted test, a clinical gold standard is used. In this case it usually means patients with a clinical picture of COVID19 in the midst of a pandemic. This isn’t an unfair assumption at all, but it is possible that other viruses may cause a very similar clinical picture to COVID19. This should, of course, cause an underestimation of the sensitivity of COVID.
Practical considerations with use of testing for physicians and patients
Everyone agrees that it is incredibly useful to know which individuals in the community have been infected by SARS-COV2. Much of the call for expansive testing capacity rests on the idea that understanding who has COVID in the community will allow for effective isolation and contact tracing to mitigate further spread of the virus. But there are some inherent limitations with testing that deserve consideration when physicians try to apply results to the patients they are treating. This also, of course, has ramifications for public health policy making. RT-PCR has limited sensitivity for the virus, and cannot be used to exclude the presence of the virus. Anywhere from 20 – 40% of patients may have COVID but be PCR negative. Serology testing, unfortunately, does not help. Serology can also yield a negative test result in an infected patient because it can take weeks to develop antibodies in the blood, and they may develop in amounts that could fall below some set threshold for detection.
Both of these tests are of limited value in the immediate diagnosis of patients where COVID-19 is suspected. RT-PCR is able to effectively rule in the presence of the virus because of its very high specificity, but serology tests unfortunately cannot. As discussed, the probability of cross-reactivity of an antibody test with non SARS-COV2 viruses raises the possibility of false positives and so have little utility in a patient population that has a low likelihood of having the infection. Even if antibodies are found, immunity to SARS-COV2 is still being understood, and it’s not known yet if the simple presence of antibodies establish immunity to the virus, or how long this may last. Other coronaviruses do show waning immunity. So RT-PCR can rule in disease, can’t rule out disease, and serologic testing can neither rule in or rule out disease.
That isn’t to say testing isn’t important. With a virus that creates as much havoc as coronavirus, testing allows society to keep track of the virus in the population generally. Think of it as a GPS that gets you to the right block, but can’t be used to get to the right house. Testing allows tracking of the rise or fall of disease spread in a population, and thus may be very important in guiding public policy but should be viewed by physicians as simply one data point to consider how best to manage a patient. Given the poor test characteristics in patients unlikely to have the disease, it’s also unclear who exactly we should be expanding testing to.
The CDC recognizes these limitations as their recommendations are to prioritize patients that have a higher risk for having COVID. It’s important to understand that this is done, not because there is limited testing capacity, but because the test result is much more useful in this population.
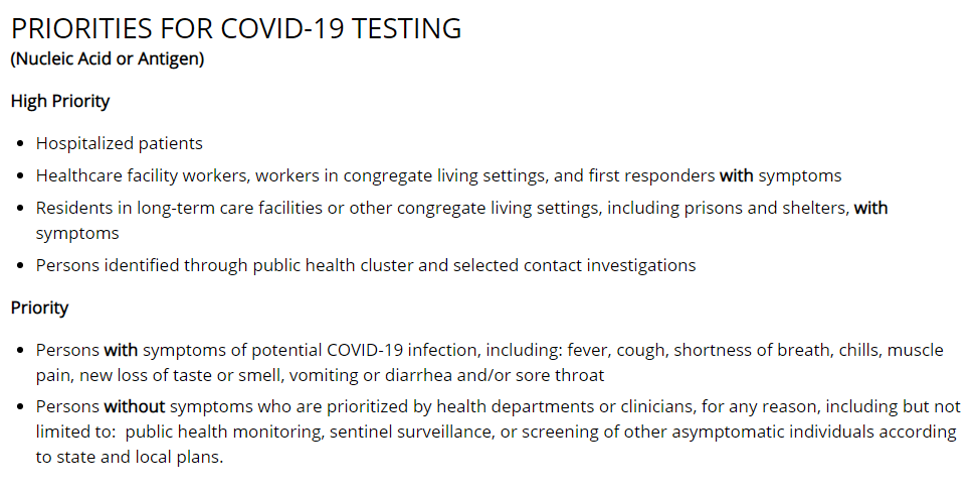
The CDC did recently update the potential symptoms from COVID-19 and in addition to the cardinal symptoms of cough and shortness of breath, added headaches. This expands the net of patients that can be tested and hopefully increases the number of patients we can capture, but realize that wider the net that’s cast with regards to symptoms that aren’t particularly specific, the lower the likelihood of disease, and the less useful the test is at predicting disease.
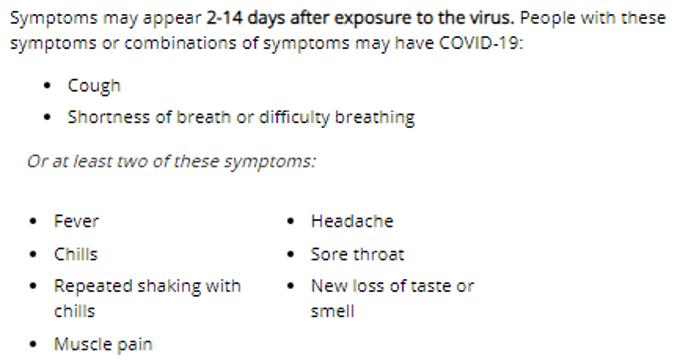
Guiding policy with testing
There are important ramifications for a number of policy prescriptions discussed. One area of active controversy relates to trying to establish the prevalence of the disease in the population. The prevalence of the disease circulation in communities is vital to understanding how useful testing is, and consequently the mitigation strategy that may need to be taken by citizens, businesses and government. The level of mitigation that is needed also depends on the perceived risk of the virus, and it’s hard to understand the risk of the virus in different communities without understanding how many people have been or are actively infected.
Attempting to establish the true prevalence of COVID has unfortunately become controversial because of the politics surrounding calculations of the fatality rate of COVID, but I’ll attempt to review some of the current data neutrally.
One study by a group of Stanford researchers looked at Santa Clara County, California. Santa Clara had the largest number of confirmed cases in any county in Northern California, and had some of the earliest known cases of COVID-19. Researchers attempted to estimate the seroprevalence in this county using targeted Facebook ads. Over 24 hours, they were able to register 3,285 adults and eventually draw blood samples from them. The test they used was a SARS-CoV-2 lateral flow assay from Premier Biotech, Minneapolis, MN. Although the test had been validated by the maker of the test (not by the FDA), the researchers sought to validate the test again in 37 samples of PCR positive COVID patients, as well as 30 pre-COVID samples from hip surgery patients. 25 of the PCR positive patients were kit positive, while 30 of the 30 pre-COVID samples were negative. They combined the validation test results provided to them from the company to arrive at a sensitivity of 80% (CI 72.1-87) and a specificity of 99.5% (CI 98.3 – 99.9).
There were 50 positive tests in the survey, which translates to a crude prevalence rate of 1.5%. Weighting their sample to match the county by zip, race and sex increases their prevalence rate to 2.8%. There is a lot of uncertainty here with the estimate because the very low numbers mean slight changes in test characteristics could completely invalidate the prevalence estimates. The authors note in their discussion that a specificity less than 97.9% would raise the possibility that all 50 positive cases were false positives. But using the current test characteristics, they estimated 48,000 and 81,000 people had been infected in Santa Clara. The number of confirmed cases at the time of testing was ~1000, suggesting the true rate of infection was 50-80x that of the confirmed cases. If that many people are infected, this suggests the risk of dying from COVID in Santa Clara is between 0.12 – 0.2 %. This very low number was the major result that many seized on. Those hoping for a quicker reopening of the economy amplified the study results, while those in favor of longer lockdowns focused on the limitations of the study. The authors were also explicit in discussing the major limitations of the study, but clearly didn’t feel that the uncertainty invalidated the results. Interestingly, a Bayesian re-analysis performed by a skeptical physician still suggested a prevalence of 20 times the reported cases. This would suggest 20,000 cases, and an infection fatality rate of 0.5%.
The Stanford group contributed to another study (not in pre-print, or peer reviewed) from Los Angeles County that suggested 4% of LA county had been infected. This suggested 221,000 to 442,000 adults in the county had been infected, an estimate that was 28 to 55 times higher than the 7,994 confirmed cases of COVID, which again pegs an Infection Fatality Rate close to 0.1-0.2%. Though these studies are an important contribution to understand seroprevalence, the small size of the studies and incompletely understood test performance results in a significant amount of uncertainty. They may reflect truth, but it’s hard to say much definitively without larger studies to further corroborate these results.
Additional data does come from the epicenter of COVID in the US is New York, which is of interest because it reflects the worst case that every other part of the country wants to avoid.
On April 23rd, Governor Cuomo of New York released data to suggest that New York City has a 20% prevalence of disease, which roughly translates to 1.7 million infected New Yorkers. As of April 23rd New York City had 151,000 cases with 16,300 deaths, which suggests an infection fatality rate of 0.9% (16,300 / 1.7 million) – a much higher estimate than the California studies, though still much lower than many have feared. Not surprisingly some attacked the number citing use of an unknown, not-yet-validated test.
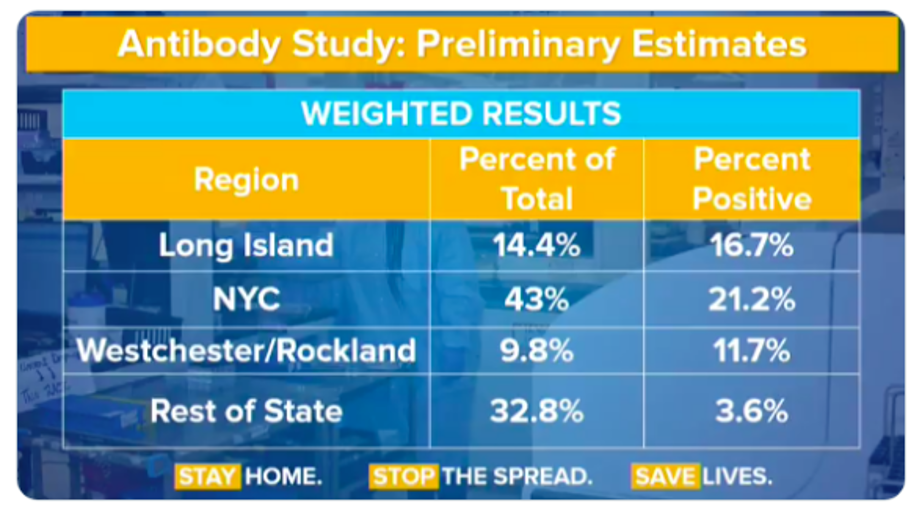
As should be clear, without knowing how well the test performs, the numbers are hard to interpret, but all is not lost. One can make assumptions and arrive at a worst case scenario. Dr. Venk Murthy – a cardiologist/researcher in Michigan – walked through a theoretical exercise on twitter that assumed all the tests done outside of the hard hit NYC metropolitan area in the state were false positives.
Governor Cuomo noted 3000 antibody tests total were done, which meant 984 (32.8% x 3000) tests were done in the rest of the state and 35 of those tests were positive (3.6% x 984). This allows a calculation of the true negatives : 984-35 = 949. Specificity can then be calculated by taking the true negatives and dividing by the total number of negative tests (sum of the true negatives and false positives.) This gives us a specificity of 96.4 % ( 949/(949+35) ). This renders a 95% confidence interval of 95.1 % -97.5 % , which is an impressively high number considering we just invalidated all positive tests in one third of the population. This is, of course, unlikely and the actual specificity is probably higher than this, meaning a 20% prevalence in New York City is indeed plausible.
Clearly, the application of widespread testing is fraught with a number of problems that has nothing to do with the number of swabs or RNA extraction kits the country may be running out of. The frustration of many public health experts has focused on the inability to ramp up testing for the virus, because it may be unsafe to open the country back up without it. The plan, as best as I can understand, is to have a vast testing capacity available to allow for the rapid isolation and quarantine of test positive citizens and their contacts. This would prevent a New York City style meltdown while keeping the economy moving in some fashion.
There are some pragmatic concerns to be raised about this plan. Seroprevalence surveys as well as observations on transmission from clinical epidemiologists seems to strongly support a virus that spreads when asymptomatic, or presymptomatic. Even if a test was 100% sensitive, if it takes 48 hours for symptoms to appear. This would require tracing back contacts over 2 days that in a major metropolitan city may run into the hundreds of individuals. The figure below is a snapshot of travelers from New York fanning out over the United States over a course of 2 days in March, after a lockdown in New York was initiated.
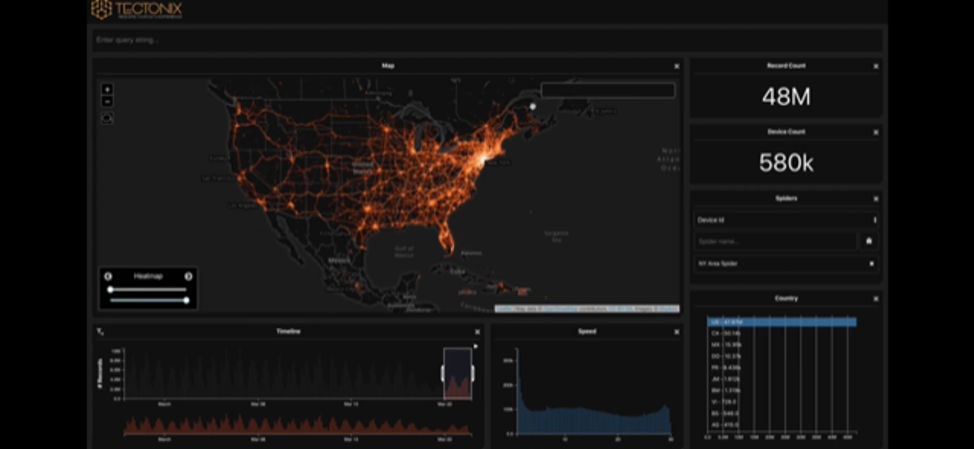
The best approach to contact tracing may piggyback off the ubiquitous smart phone and make use of bluetooth signals given off to allow for a time and location based map of every phone a positive patient’s phone came into contact with. But this plan has some holes in it as well. For it to work, it would require about 60% of the population to install a contact tracing app. Even in non-libertarian, beat-you-with-a-stick-for-chewing-gum rule following Singapore, this number is closer to 12%. The other big boring problem is false positives. Bluetooth goes through walls, so you could register a contact even if you were on either side of a wall, or if someone jogged by you while 6 feet away. The application of technology from Silicon Valley has a cool factor that seems to mesmerize large swaths of the public and government officials into sending lots of taxpayer dollars for solutions that don’t work well. But being cool shouldn’t absolve technology from demonstrating robust outcomes or at least robust plausibility prior to buy in from the taxpayer. It’s also notable that Singapore, a very small country with a population of 5 million, is in the midst of a significant outbreak that forced a widespread lockdown despite its widely praised and ballyhooed app-based contact tracing.
Layer this on top of tests that aren’t effectively able to rule out the presence of disease, and you have a very high mountain to climb to make this all work well.
Consider the employees of a small doctor’s office. Should asymptomatic employees be tested? Given the low positive predictive value in low risk patients, does a positive antibody test in an employee in this setting be reassuring? How often do employees need to miss work to get tested? Should we be running routine surveillance for active infection with RT-PCR? Since PCR only tells us if there’s an infection at the time of the test, how often should we be testing with PCR? If one employee does test positive, does the whole office need to shut down?
The availability of widespread testing for symptomatic patients appears to be of paramount importance, not so much because it can effectively rule out disease, but because it can capture new outbreaks of disease in a population. Scaling testing to the point we can make decisions for the individual based only on the results of the test seems to be beyond the scope of current testing technology.
The only evidence to support the efficacy of widespread testing seems to derive from the general idea that countries that test a lot have the lowest death rates. One such chart making the rounds is below.
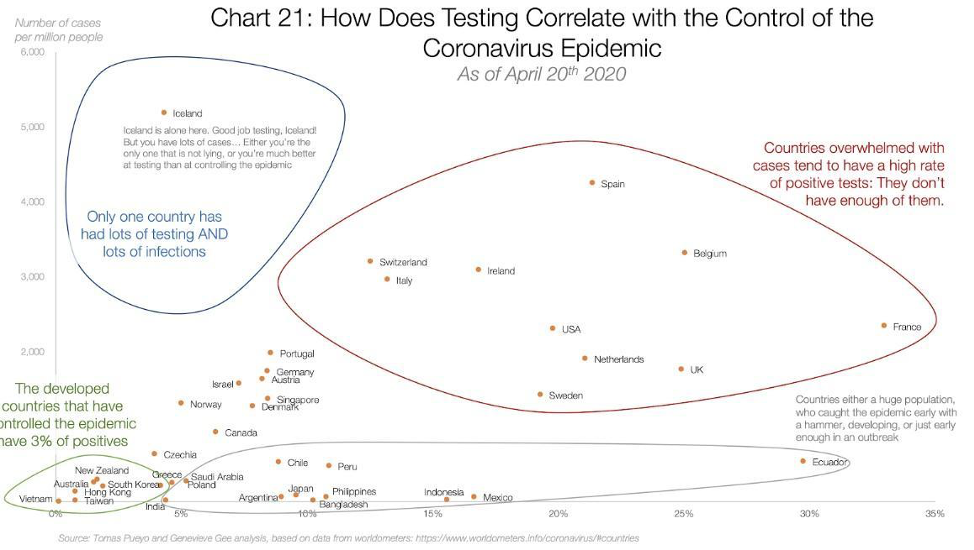
The circles may seem compelling to some, but beyond the foolishness of confusing correlation and causation, even correlation is hard to find when you try to draw a line through these data points. Figures below provided by Andrew Foy, (Cardiologist, Hershey, PA) demonstrates no correlation between amount of testing and death rate.
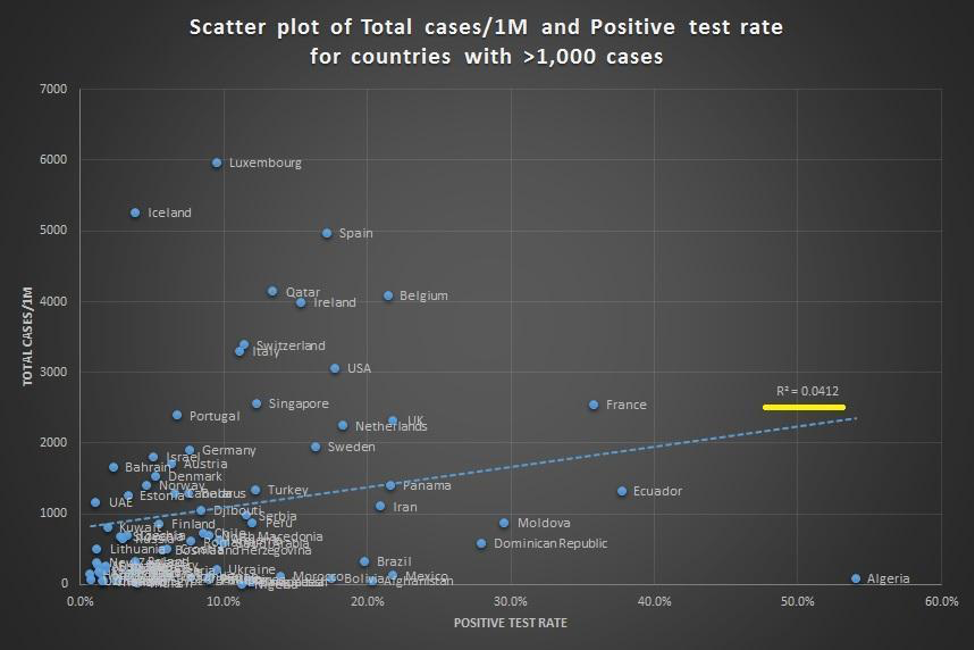
This should not be surprising given that the testing hypothesis papers over the vast differences in countries with regards to size, density, geographic location, demographic, connectedness and highly variable policies related to travel and social distancing. It also fails to appreciate differences in testing availability, and assumes COVID related death counts are accurate everywhere. The certainty in some quarters that testing will be some panacea is especially surprising given the opprobrium from the same quarters for strong conclusions by Stanford researchers from the early serologic prevalence data.
Conclusions
- Testing is important to track the trajectory of an epidemic in a community to guide local or national efforts at mitigation
- The tests we currently have for COVID have limited accuracy for the individual patient
- Antibody testing suggests that the fatality rate for COVID may be low in certain communities, but data from New York suggests there is the potential for significant death and morbidity in any major metropolitan area
- Contact tracing enabled by smart phone technology is likely unable to be effective because they do not overcome the inherent limitations of COVID testing, require widespread adoption, and may not even be accurate at determining high risk contacts.
- Mass testing alone is unlikely to completely explain the varying death rates seen by country
- Testing is no panacea. This suggests a comprehensive strategy that incorporates testing, but doesn’t rely on it.
Anish Koka is a Cardiologist in practice in Philadelphia. He is co-host of the podcast, The Accad & Koka Report, and can be followed on Twitter @anish_koka. An edited version of this piece appeared in Medscape.
Categories: Uncategorized
Very good article. An exhaustive review of the statistical science related to testing. I learned a hell of a lot.
The accuracy of serological testing for COVID-19 antibodies could be improved significantly by using two antibody tests , instead of just relying on a single test.
Take the example with the population prevalence at 10%. A test with 90% sensitivity and 95% specificity gives the PPV 66.7% or 33.3% false positive. The PPV will increase to 97.3% or 2.7% false positive if two test approach is used.
Though it is certain that the prevalence of the disease influences the chances of a correct test result, doing a repeated test can reduce the chance of having false positives.