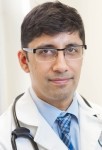
By ANISH KOKA MD
The day after NBC releases a story on a ‘ground-breaking’ observational study demonstrating caramel macchiatas reduce the risk of death, everyone expects physicians to be experts on the subject. The truth is that most of us hope John Mandrola has written a smart blog on the topic so we know intelligent things to tell patients and family members.
A minority of physicians actually read the original study, and of those who read the study, even fewer have any real idea of the statistical ingredients used to make the study. Imagine not knowing whether the sausage you just ate contained rat droppings. At least there is some hope the tongue may provide some objective measure of the horror within.
Data that emerges from statistical black boxes typically have no neutral arbiter of truth. The process is designed to reveal from complex data sets, that which cannot be readily seen. The crisis created is self-evident: With no objective way of recognizing reality, it is entirely possible and inevitable for illusions to proliferate.
This tension has always defined scientific progress over the centuries as new theories overturned old ones. The difference more recently is that modern scientific methodology believes it possible to trade in theories for certainty. The path to certainty was paved by the simple p value. No matter the question asked, how complex the data set was, observational or randomized, p values < .05 mean truth.
But even a poor student of epistemology recognizes that all may not be well in Denmark with regards to the pursuit of truth in this manner. Is a p value of .06 really something utterly different from a p value of .05? Are researchers bending to the pressures of academic advancement or financial inducements to consciously or unconsciously design trials that give us p values <.05?
The slow realization the system may not be working comes from efforts to replicate studies. Methodologist guru Brian Nosek convinced 270 of his psychology colleagues in 2015 to attempt to replicate 100 prior published trials. Only 36% of the studies gave the same result as the original. Imagine the consternation if an apple detaching from a tree only fell to the ground 36% of the time.
Why this is happening is a fascinating question that forms the subject of Nosek’s most recent published paper that focuses on the statistical black box data is fed into.
29 statistical teams aggregated via Twitter were given one complex dataset and tasked with finding out if football player skin tone had anything to do with referees awarding red cards. The goal was to put the statistical methods to the test. If you give the same question and data to 29 different teams, does the analysis result in the same answer?
In the forest plot summarizing the findings, the results of the 29 teams do not, at first glance, appear to be remarkably different. The majority of teams get the same qualitative answer by being on the ‘right’ side of the magical p of 0.05 threshold, though I imagine the vast number of consumers of medical evidence would be surprised to find that depending on the statistical model employed, the likelihood of the sky being blue is ~70%. More discriminating readers will ignore the artificial cliff dividing blue from not blue to point out the wide overlap in confidence intervals that suggest the same basic answer was arrived at with minimal beating around the bush.
But a review of the meticulous steps taken by the project managers of the study demonstrate the convergence of the results is somewhat of an engineered phenomenon. After collection of the data set and dissemination of the data to the statistical teams, the initial approaches the teams took were shared among the group. Each team then received feedback on their statistical approach and had the opportunity to adjust their analytic strategy. Feedback incorporated, the teams ran the data through their selected strategies, and the results produced were again shared among all the teams.
The idea of the various steps taken, of course, was not to purposefully fashion similar outputs for the trial, but to simulate a statistically rigorous peer review that I’m told is rare for most journals. Despite all the feedback, collaboration and discussion, 29 teams ended up using 21 unique combinations of co-variates. Apparently statisticians choosing analytic methods are more Queer Eye for the Straight Guy, less HAL. Sometimes the black pants go with that sequin top, other nights only the feather boa completes the outfit.
The findings were boring to most statisticians, but titillating to most clinicians. The statistical criticism is a little unfair. It is certainly true that the problem of analysis-contingent results isn’t completely novel. Simonsohn et. al. use the phrase p-hacking to describe unethical researchers throwing line after line into a dataset to find statistically significant associations.
Gelman and Lokens argue this is a simplistic frame that describes the minority of researchers. What they believe to be far more common and concerning are researchers embarking on projects with strong pre-existing biases consciously or unconsciously choosing analytic paths that end up confirming their biases. This problem has been attractively described as the garden of forking paths.
The current project fits into neither one of these buckets. The researchers had no incentive to get a statistically significant result because publishing wasn’t dependent on getting a p < .05. And this particular data set had a limited number of forking paths to traverse because the question asked of the data set was specific – red cards and skin tone. The teams couldn’t choose to look at the interaction of yellow cards and GDP of player home countries, for instance. And perhaps most importantly, the teams were not particularly motivated to arrive at an answer as confirmed by a survey completed at the start of the trial.
Implications of this study loom especially large for healthcare, where policy making has so far been the provenance of enlightened academics who believe a centrally managed well-functioning technocracy is the best way to manage the health needs of the nation.
The only problem is that the technocrats have so far excelled mostly at failing spectacularly. Public reporting of cardiovascular outcomes was supposed to penalize poor performers, and reward those that excelled. Instead, it resulted in risk aversion by physicians which meant fewer chances for the sickest patients who most needed help. The Hospital Readmission Reduction Program (HRRP) was supposed to focus the health system on preventable readmissions. The health system responded by decreasing readmissions at the expense of higher mortality.
One of the problems with most health policy research – highlighted in a recent NEJM perspective – is that it largely rests on analyses of observational data sets of questionable quality. What isn’t mentioned is that the conclusions made about policy can depend on who you ask.
This won’t surprise Andrew Gelman or Brian Nosek, but the health policy researchers responsible for devising the HRRP program publishes repeatedly in support of their stance that reduced admissions as a consequence of the program is not correlated with higher heart failure mortality, while cardiologists who take care of heart failure patients produce data that traces heart failure mortality to initiation of the HRRP program. Who to believe?
In their NEJM perspective, Bhatt, and Wadhera don’t mention this divide, but do call for better research that will migrate the health care landscape from “idea based policy” to “evidence based policy”. The solutions lie in natural randomized trials, and where the data sets won’t comply, use the $1 billion/year budget of the Center for Medicare and Medicaid Innovations (CMMI) to run mandatory policy RCTs in small groups before broad rollout of policy to the public. This perspective is as admirable as it is short sighted and devoid of context.
Randomized control trials are difficult to do in this space. But even if RCTs could be done, would it end debate? RCTs may account for covariates but, as discussed, this is just one source of variation when analyzing data. Last I checked, cardiologists with the benefit of thousands of patients worth of RCTs continue to argue about statins, fish oil, and coronary stents, and these areas are completely devoid of political considerations.
The Oregon experiment, one of the largest, most rigorous RCTs of Medicaid expansion, hasn’t ended debate between conservatives and liberals on whether the nation should expand health coverage in this fashion. And nor should it. Both sides may want to stop pretending that the evidence will tell us anything definitively. Science can tell us the earth isn’t flat, it won’t tell us if we should expand Medicaid.
Evidence has its limits. Health care policy research for now remains the playground of motivated researchers who consciously, or unconsciously produce research confirming their biases. Indeed, the mistake that has powered a thousand ProPublica articles on conflict of interest isn’t that financial conflicts aren’t important, it’s that concentrating on only one bias is really dumb.
And Nosek’s team clearly demonstrates that even devoid of bias, a buffet of results are bound to be produced with something palatable for every ideology. The path forward suggested by some in the methodologist community involves crowd-sourcing all analysis where possible. While palate pleasing, this seems an inefficient, resource heavy enterprise that still leaves one with an uncertain answer.
I’d settle for less hubris on the part of researchers who would seem to think an answer lives in every data set. Of the 2,053 total players in Nosek’s football study, photographs were only available for 1500 players. No information was available on referee skin tone – a seemingly relevant piece of data when trying to assess racial bias.
Perhaps the best approach to certain research questions is to not try to answer them. There is no way to parse mortality in US hospitals on the basis of physician gender, but someone will surely try and, remarkably, feel confident enough to attach a number to the thousands of lived saved if there were no male physicians.
If the point of applying empiricism to the social sciences was to defeat ideology with a statistically powered truth machine, empiricism has fallen well short. Paradoxically, salvation of the research enterprise may lie in doing less research and in imbuing much of what’s published with the uncertainty it well deserves.
Anish Koka is a cardiologist in private practice in Philadelphia. He can be followed on Twitter @anish_koka. This post originally appeared here on The Accad & Koka Report.
Categories: Uncategorized
workout with health and fitness app!
Health,fitness & diabetic control
Health,fitness
Health,fitness & diabetic control
This is helpful in getting a gut feeling of the meaning of the p-value… which essentialky says: A claim is assumed valid if its counterclaim is improbable.
Even without much mathematics acumen we can easily see holes in this logic. The claim itself–called the null hypothesis–could be nutty. Or its distribution could be crazy and not “normal”. And whether the counterclaim (the p value of it) turns out to be highly improbable* or highly probable can leave us with no meaning at all if the original claim–the null hypothesis–is kooky.
*<.05 is the usually used value
The rumor spread by word-of-mouth. Eventually, the subject of the rumor was quoted as saying “The notice of my demise is greatly exaggerated.” Even this widely acknowledged quotation of Mark Twain, aka Samuel Clemens, has now been described as partially false.
As per this post, I also note the findings of a study published in the first report (2018) of THE MILBANK QUARTERLY by Lawton R. Burns and Mark V. Pauly from The Wharton School, University of Pennsylvania. Its title is: “Transformation of the Health Care Industry: Curb your enthusiasm?” The study covered an extended period of time and assessed the attributes of published studies for the various models of PPOs. I quote the final two sentences of the “Findings” section: “Data suggest a low prevalence of provider risk payment models and slow movement toward new payment and organizational models. Evidence suggests the impact of both on cost and quality is weak.” For their “Policy Points” section, the last sentence states: “In the longer run, decision makers should be prepared to accept the limits on transformation and carefully consider whether to advocate solutions not yet supported by evidence.”
All of this is likely on autopilot because of the Paradigm Paralysis that pervades the large institutional enterprises that dominate our nation’s healthcare industry. This Paradigm Paralysis began as a result of the institutional codependency that exists between the providers of Complex Healthcare and the institutions that pay for this Complex Healthcare. The Paradigm Paralysis has continued as the providers and payers have focused on their market share priorities as opposed to their Social Responsibilities. The principal enablers are the Federal government and the electronic health record industry.
Contributing to the Paradigm Paralysis is a lack of understanding that a major cause of the cost and quality problems is driven by the decline of our nation’s level of Social Capital community by community. It is most poignantly measurable by the loss of Social Mobility within the lowest income level of citizens. It has fallen from 90% in 1940 to near 00% by @1970. Social Mobility measures the likelihood that a person has a higher income as an adult than his parents earned when he was a child. The future loss of hopefulness for a person’s “Well-Being” is eventually catastrophic.
HINT: The loss of Social Capital and its implications, as measured by Social Mobility, cannot be fixed with another governmental hand-out. The following quotation from Eleanor Roosevelt applies: “Its better for every body when it gets better for everybody.” I offer my own definition for SOCIAL CAPITAL.
.”A community’s norms of Trust, Cooperation and Reciprocity that
.its citizens will more commonly apply for resolving the Social Dilemmas
.they encounter daily within their community’s Municipal Life
.WHEN Caring Relationships increasingly pervade
.the social networks of the community’s citizens,
.especially the enduring Caring Relationships portrayed
.within the Micro-Neighborhood Network of each citizen’s Family.”
For Burns and Pauly https://doi.org/10.1111/1468-0009.12312