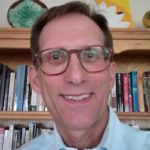
BY KIM BELLARD
As many of you did, I followed the recent debt ceiling saga closely, and am relieved that we now have a compromise, of sorts. The House Republicans demanded a lot of things, most of which they did not get, but one area where they did prevail was in toughening work requirements for food (SNAP) and income (TANF). They somehow believe that there are uncounted numbers of “able-bodied” people sitting around on their couches collecting government benefits, a myth that goes back to Ronald Reagan’s welfare queen stereotype, and have long advocated work requirements as the remedy.
Ironically, according to the CBO, the work requirements passed may actually increase federal spending by as much as $2b, and increase the number of monthly recipients by as many as 80,000 people, but who’s counting?
All this seems timely because of some new studies that illustrate – once again — that, yes, poverty is bad for people’s health, and helping them get even a little bit more out of poverty improves their health.
———–
The first study, by Richterman, et. alia, looked at the impacts of cash transfers – i.e., giving poor people money with little or no strings attached – had on adult and child mortality. I might parenthetically add that the study looked at programs in low and middle income countries, to which some might argue that, well, America is neither, so the results don’t apply.
To which I would counter: umm, have you looked at the percent of Americans below the poverty line? Have you looked at the number of Americans who go hungry every night? Have you looked at our mortality rates, especially for maternal and infant mortality? Have you looked at health statistics for disadvantaged populations in the U.S.? No doubt: we’ve got low and middle income colonies within our country, to co-opt the title of Chris Hayes’ searing book on criminal justice in America.
Dr. Richterman and his colleagues looked at longitudinal data on some 7 million individuals – 4 million adults, 3 million people – from 29 countries that had cash transfer programs. The transfers were often small, sometimes as little as $100, but the impacts were not. The result:
Our primary finding was that these programmes were associated with significant mortality reductions among women and children aged less than 5 years, indicating the important role that these anti-poverty initiatives have had in promoting population health over the last 20 years.
The mortality reductions were on the order of 20% for women and 8% for children under 5, and grew over time.
Importantly, the effects were greatest among populations that started with the lowest health spending and shortest life expectancies. The largest impacts were among women; the authors note: “This adds to previous evidence that cash transfers may disproportionately benefit women, or be more effective when women are the primary beneficiaries.”
Critics fear that such payments might be misused – e.g., buying junk food, drugs, or alcohol – but Audrey Pettifor, a social epidemiologist at the University of North Carolina who studies such things (but was not involved in this research), told The New York Times: “the data just doesn’t back that up.”
The second study, by Dillman et. alia, in NEJM Catalyst, looked directly at SNAP; specifically, the impact on enrollment in SNAP had on health and cost outcomes. It looked at health care utilization (inpatient hospitalization, ED visits, and unplanned care) and health care costs (medical, pharmacy, and total cost of care) during the first two years of having SNAP, versus a matched comparison group without SNAP.
Again, the results were striking: SNAP enrollees had 16% lower medical costs and 21% lower pharmacy costs in their first year of enrollment, and 16%/20% lower costs in the second year. That’s remarkable.
If, for example, you had responsibility for a state Medicaid program, you might want to think about that when your Representatives and/or state legislators starts talking about restricting SNAP benefits.
The third study, by Pollack et. alia, in JAMA Network, looked what happens to asthma morbidity among children when their families get the opportunity to move from disadvantaged, low income, urban neighborhoods to low poverty neighborhoods. Asthma is known to be more prevalent in such high poverty neighborhoods, due to sub-standard housing stock and other environmental factors.
The findings: asthma flare-ups (“exacerbations”) dropped by more than half (0.88 per person year to 0.40) after such a move. The authors further noted: “Measures of stress, including social cohesion, neighborhood safety, and urban stress, all improved with moving and were estimated to mediate between 29% and 35% of the association between moving and asthma exacerbations.”
One might reasonably speculate that the impact on other health conditions – e.g., COPD, diabetes, obesity, gun violence, drug overdoses – would show similarly positive results as well.
—————-
The New Yorker recently profiled public health researcher Arline T. Geronimus, whose new book introduces us to a word I wish we never had to think about: Weathering: The Extraordinary Stress of Ordinary Life in an Unjust Society. “Weathering” in this context refers to the cumulative stress of racial and economic inequality, such as inadequate medical care, hazardous living environments, or insufficient diet.
The result, Professor Geronimus found, is that “people who endure chronic stressors and other forms of structural violence can biologically age faster than their peers.” Thus the various disparities in morbidity and mortality that we see – and that cash transfer programs can help ameliorate.
If all this sounds like I’m arguing for guaranteed income programs, it’s because I am. I’ve written about universal income programs (UBI) and baby bonds, because what we’re doing now is not working. The COVID relief payments, which were perhaps the closest the U.S. has come to UBI, may now be viewed by some conservatives as wasteful spending, but it had “historic” impacts on lifting more families out of poverty.
I’m thrilled that there are as many as 130 guaranteed income programs, in places like Stockton (CA), Atlanta, Austin, Chicago, Jackson (MS), Long Beach, NYC, Philadelphia, and St. Paul (MN), and the evidence is that such programs work. But they’re still too little, impacting too few.
If certain politicians can’t get past their “moral” or political principles against guaranteed income, perhaps the above studies might help convince them that the improvements in health/health spending such programs bring makes them in everyone’s best fiscal interests.
The New Yorker article quotes Dr. Vicente Navarro, a professor emeritus of health and public policy at Johns Hopkins University:, “It is not inequalities that kill people. It is those who are responsible for these inequalities that kill people.”
We are those people. We can do better.
Kim is a former emarketing exec at a major Blues plan, editor of the late & lamented Tincture.io, and now regular THCB contributor
Categories: Health Policy
Statistical analysis is the most effective tool we have to correlate data to root causes; it is arguably the only effective way to do so.
Unfortunately, as in this case, most analysis that makes it into media publication is flawed. I do not argue with the opinions… but they cannot simply be assumed to be correct nor to be supported by the data.
This author made 3 critical errors:
1) Garbage in always yields garbage out. Drawing conclusions from data drawn from one population and then extending it to others simply based on opinions, even those of experts, is questionable at best. An example,
“One might reasonably speculate that the impact on other health conditions – e.g., COPD, diabetes, obesity, gun violence, drug overdoses – would show similarly positive results as well.”
The author, to his credit, acknowledges the speculative nature of this particular statement, but it is simply serves to expand the narrative beyond the scope of the data.
2) Quoting percentages without their basis is a frequently used way to lie with statistics. Media uses this technique relentlessly to mislead their audiences. A 90% reduction in anything is meaningless when based on a small base. This isn’t about the sample size or the confidence levell, it’s about the magnitude of the denominator in the percentage calculation. For example… several years ago a newly appointed FFA director set one of the safety improvement goal at a 50% reduction of in-flight passenger injuries. Sounds great, but as one of the audience pointed out, only 4 people of the millions who flew the prior year had been injured! Meeting the lofty sounding goal would effectively reduce the injuries from 4 to 2.
3) The single most important use of statistical analysis is to identify root causes. The statistics is really the easy part… it’s a well defined mathematical tool so the analysis is usually rigorous. Finding the actual root cause requires real work. This is where the wheat must be separated from the chaff. It requires multiple iterations by a group of interested persons (not just “experts”). Multiple potential causes must be evaluated; concensus must be reached; selected causes must be validated with targeted testing; a root cause selected; a potential solution to it must be defined and tested. One popular method to do all this is Six Sigma. Developed 25 years ago for manufacturing processes it’s been successfully used in virtually all disciplines. The steps are Define, Measure, Analyze, Improve, and Control (DMAIC).
When leading their audience to a conclusion the media has a responsibility to present opinion as as opinion. Getting folks to take action is an important duty of all media. It’s a much more difficult job than just quoting data to support a position, even one that may be correct.
Opinions and rhetoric are thrown at the public from all directions. No question the media has a tough job to do… but the public relies on them for the truth. Before leaping to a solution to such an important issue it’s best to follow Reagan’s recommendation to “Trust, but verify”.