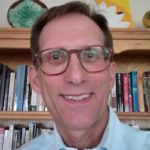
BY KIM BELLARD
If you’ve been following artificial intelligence (AI) lately – and you should be – then you may have started thinking about how it’s going to change the world. In terms of its potential impact on society, it’s been compared to the introduction of the Internet, the invention of the printing press, even the first use of the wheel. Maybe you’ve played with it, maybe you know enough to worry about what it might mean for your job, but one thing you shouldn’t ignore: like any technology, it can be used for both good and bad.
If you thought cyberattacks/cybercrimes were bad when done by humans or simple bots, just wait to see what AI can do. And, as Ryan Health wrote in Axios, “AI can also weaponize modern medicine against the same people it sets out to cure.”
We may need DarkBERT, and the Dark Web, to help protect us.
A new study showed how AI can create much more effective, cheaper spear phishing campaigns, and the author notes that the campaigns can also use “convincing voice clones of individuals.” He notes: “By engaging in natural language dialog with targets, AI agents can lull victims into a false sense of trust and familiarity prior to launching attacks.”
Continue reading…